Quote from
ephraim on October 28, 2023, 10:02 pm
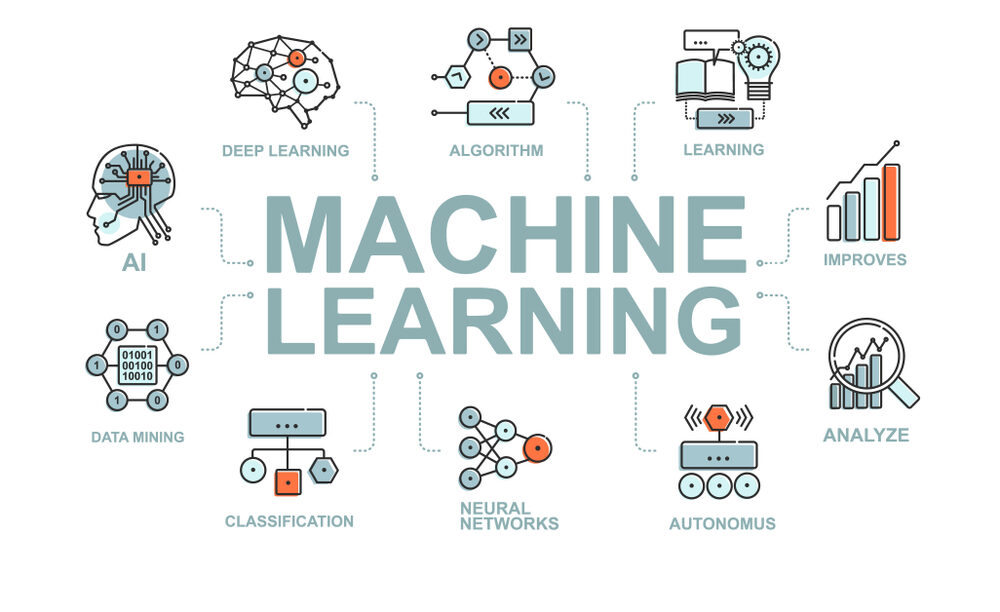
Machine Learning (ML) is not just a buzzword; it's a transformative technology that's reshaping our world. In this post, let's embark on a journey into the captivating realm of Machine Learning to understand what it is, its applications, and why it's the driving force behind many cutting-edge innovations.
What is Machine Learning?
At its core, Machine Learning is a subset of artificial intelligence (AI) that empowers computer systems to learn from data and improve their performance without being explicitly programmed. In essence, machines "learn" by recognizing patterns in data and making predictions or decisions based on those patterns.
Types of Machine Learning:
-
Supervised Learning: In this type, models are trained on labeled data, where the input and desired output are known. It's widely used in tasks like classification and regression.
-
Unsupervised Learning: Here, models work with unlabeled data, discovering patterns or relationships within the data. Clustering and dimensionality reduction are common applications.
-
Reinforcement Learning: This approach involves training models to make sequences of decisions in an environment to achieve a goal. It's employed in robotics, gaming, and autonomous systems.
-
Semi-Supervised Learning: A blend of supervised and unsupervised learning, it uses a small amount of labeled data alongside a more extensive unlabeled dataset.
-
Deep Learning: A subset of ML, deep learning employs neural networks with many layers (deep neural networks) to process and interpret data, particularly in tasks like image and speech recognition.
Applications of Machine Learning:
Machine Learning has penetrated numerous aspects of our lives and industries:
-
Healthcare: ML assists in early disease detection, personalized treatment plans, and drug discovery.
-
Finance: It's used for fraud detection, algorithmic trading, risk assessment, and credit scoring.
-
E-commerce: ML powers recommendation engines, chatbots, and demand forecasting.
-
Autonomous Vehicles: In self-driving cars, ML helps process sensor data to navigate safely.
-
Natural Language Processing (NLP): ML enables language translation, sentiment analysis, and virtual assistants like Siri and Alexa.
-
Image and Video Analysis: It's employed in facial recognition, object detection, and content moderation.
Why Machine Learning Matters:
-
Data-Driven Decision Making: ML enables businesses and organizations to extract valuable insights from vast datasets, aiding in informed decision-making.
-
Automation: It automates tedious and repetitive tasks, freeing up human resources for more creative and strategic endeavors.
-
Personalization: ML powers personalized content recommendations, making our online experiences more relevant and engaging.
-
Efficiency and Accuracy: It can process data faster and with greater accuracy than humans, leading to enhanced productivity.
-
Innovation: ML is at the forefront of technological advancements, from AI-driven art to medical breakthroughs.
Challenges and Considerations:
While the potential of Machine Learning is immense, it's not without challenges. Ensuring data privacy, addressing bias in algorithms, and understanding the ethical implications of AI are critical considerations. Proper training data, computational resources, and domain expertise are also essential for successful ML implementations.
In conclusion, Machine Learning is the driving force behind many technological wonders of our time. Its applications are diverse, and its potential is limitless. As we venture further into the future, embracing and understanding this transformative technology will be key to staying at the forefront of innovation.
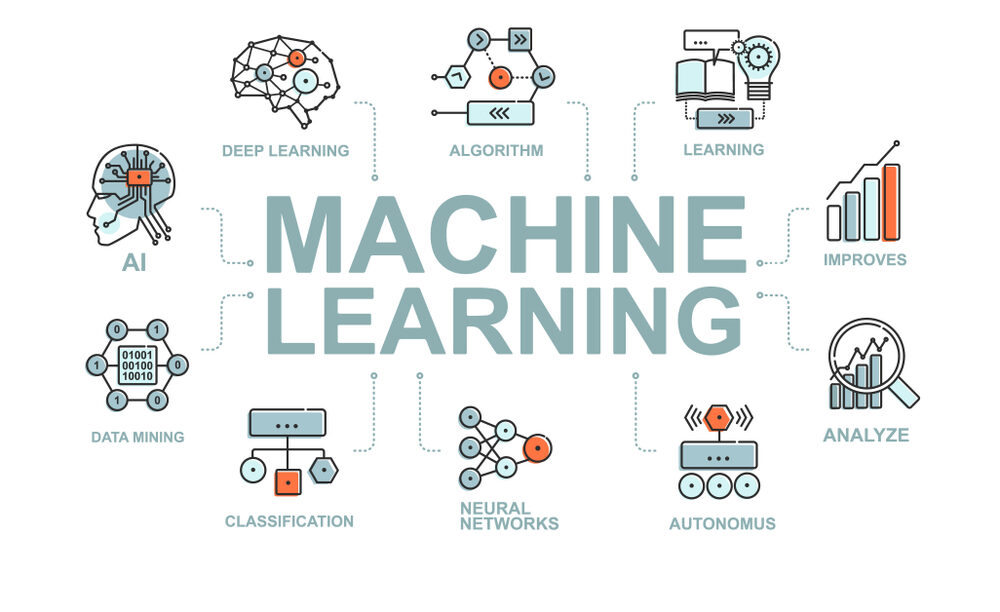
Machine Learning (ML) is not just a buzzword; it's a transformative technology that's reshaping our world. In this post, let's embark on a journey into the captivating realm of Machine Learning to understand what it is, its applications, and why it's the driving force behind many cutting-edge innovations.
What is Machine Learning?
At its core, Machine Learning is a subset of artificial intelligence (AI) that empowers computer systems to learn from data and improve their performance without being explicitly programmed. In essence, machines "learn" by recognizing patterns in data and making predictions or decisions based on those patterns.
Types of Machine Learning:
-
Supervised Learning: In this type, models are trained on labeled data, where the input and desired output are known. It's widely used in tasks like classification and regression.
-
Unsupervised Learning: Here, models work with unlabeled data, discovering patterns or relationships within the data. Clustering and dimensionality reduction are common applications.
-
Reinforcement Learning: This approach involves training models to make sequences of decisions in an environment to achieve a goal. It's employed in robotics, gaming, and autonomous systems.
-
Semi-Supervised Learning: A blend of supervised and unsupervised learning, it uses a small amount of labeled data alongside a more extensive unlabeled dataset.
-
Deep Learning: A subset of ML, deep learning employs neural networks with many layers (deep neural networks) to process and interpret data, particularly in tasks like image and speech recognition.
Applications of Machine Learning:
Machine Learning has penetrated numerous aspects of our lives and industries:
-
Healthcare: ML assists in early disease detection, personalized treatment plans, and drug discovery.
-
Finance: It's used for fraud detection, algorithmic trading, risk assessment, and credit scoring.
-
E-commerce: ML powers recommendation engines, chatbots, and demand forecasting.
-
Autonomous Vehicles: In self-driving cars, ML helps process sensor data to navigate safely.
-
Natural Language Processing (NLP): ML enables language translation, sentiment analysis, and virtual assistants like Siri and Alexa.
-
Image and Video Analysis: It's employed in facial recognition, object detection, and content moderation.
Why Machine Learning Matters:
-
Data-Driven Decision Making: ML enables businesses and organizations to extract valuable insights from vast datasets, aiding in informed decision-making.
-
Automation: It automates tedious and repetitive tasks, freeing up human resources for more creative and strategic endeavors.
-
Personalization: ML powers personalized content recommendations, making our online experiences more relevant and engaging.
-
Efficiency and Accuracy: It can process data faster and with greater accuracy than humans, leading to enhanced productivity.
-
Innovation: ML is at the forefront of technological advancements, from AI-driven art to medical breakthroughs.
Challenges and Considerations:
While the potential of Machine Learning is immense, it's not without challenges. Ensuring data privacy, addressing bias in algorithms, and understanding the ethical implications of AI are critical considerations. Proper training data, computational resources, and domain expertise are also essential for successful ML implementations.
In conclusion, Machine Learning is the driving force behind many technological wonders of our time. Its applications are diverse, and its potential is limitless. As we venture further into the future, embracing and understanding this transformative technology will be key to staying at the forefront of innovation.